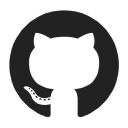
23 Sep 2022
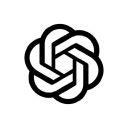
Paper: Robust Speech Recognition via Large Scale Weak Supervision
Model Card (?):
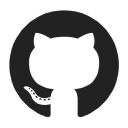
3rd Party Resources

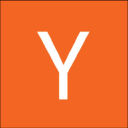
Getting Started
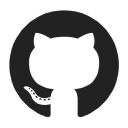
install
pip3 install git+https://github/openai/whisper.git
usage
Working 1st try:
- in less than 3mns
- with a .m4a
file (requiring no conversion to .wav
)
- with 4 lines of code (!)
- with very good accuracy
🤯 😁
import whisper
model = whisper.load_model("base")
result = model.transcribe('test/1.m4a')
print(f'\n{result["text"]}\n')
What is missing for voice transcripts, is voice commands recognition, eg "comma", "new line", "full stop", etc..
I can add those in my script though.
25 Sep 2022
It also detects language automatically, and pretty accurately so far!
response structure
{ 'language': 'en',
'segments': [ { 'avg_logprob': float,
'compression_ratio': float,
'end': float,
'id': int,
'no_speech_prob': float,
'seek': int,
'start': float,
'temperature': float,
'text': str,
'tokens': [ int,
int,
int,
...,
]}],
'text': str # returned transcript
}
example:
file = 'test/test_from_watch.m4a'
import whisper
model = whisper.load_model("base")
result = model.transcribe(file)
pp.pprint(result)
outputs:
UserWarning: FP16 is not supported on CPU; using FP32 instead
warnings.warn("FP16 is not supported on CPU; using FP32 instead")
Detected language: english
{ 'language': 'en',
'segments': [ { 'avg_logprob': -0.4222838251214278,
'compression_ratio': 0.9866666666666667,
'end': 11.200000000000001,
'id': 0,
'no_speech_prob': 0.11099651455879211,
'seek': 0,
'start': 0.0,
'temperature': 0.0,
'text': ' This is a test of a transcript in English '
'recorded from my watch fullstop.',
'tokens': [ 50364,
639,
307,
257,
1500,
295,
257,
24444,
294,
3669,
8287,
490,
452,
1159,
1577,
13559,
13,
50924]}],
'text': ' This is a test of a transcript in English recorded from my watch '
'fullstop.'}
Note: need to figure out what the UserWarning
is about 🤔
26 Sep 2022
working really well in implementation now in use of Transcribee: Transcribee
testing for meeting recordings
would be great to have a timed transcript, similar to a subtitle file.
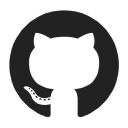
found:
# Write a transcript to a file in SRT format.
# Example usage:
from pathlib import Path
from whisper.utils import write_srt
result = transcribe(model, audio_path, temperature=temperature, **args)
# save SRT
audio_basename = Path(audio_path).stem
with open(Path(output_dir) / (audio_basename + ".srt"), "w", encoding="utf-8") as srt:
write_srt(result["segments"], file=srt)
from: https://github/openai/whisper/blob/main/whisper/utils.py
Newest version includes a write_srt
function.
Need to update
Configuration options
2025-01-06 06:05 (to test)
print(f"Loading audio file: {args.audio_path}")
audio = whisper.load_audio(args.audio_path)
# Load the Whisper model
print(f"Loading Whisper model: {args.model_size} on device '{final_device}'")
model = whisper.load_model(args.model_size, device=final_device)
# Configuration for transcription
transcription_config = {
"language": "en", # Force English transcription
"task": "transcribe", # Speech recognition (not translation)
"vad": "silero", # Use Voice Activity Detection to remove non-speech segments
"detect_disfluencies": True, # Detect and include disfluencies
"trust_whisper_timestamps": True, # Trust Whisper's native timestamps
"compute_word_confidence": True, # Compute word-level confidence scores
"include_punctuation_in_confidence": True, # Punctuation confidence
"min_word_duration": 0.1, # Minimum duration for word timestamps
"plot_word_alignment": False, # Disable plotting alignment
"compression_ratio_threshold": 2.4, # Avoid highly repetitive outputs
"logprob_threshold": -1.0, # Filter low-confidence outputs
"no_speech_threshold": 0.6, # Adjust silence sensitivity
"beam_size": 5, # Beam search size
"best_of": 5, # Consider top candidates
"temperature": (0.0, 0.2, 0.4, 0.6, 0.8), # Fallback decoding temps
"condition_on_previous_text": True, # Context-aware transcription
"refine_whisper_precision": 0.02, # Precision for refining timestamps
"remove_empty_words": True, # Remove empty words
}
# Transcribe the audio
print("Transcribing audio...")
result = whisper.transcribe(model, audio, **transcription_config)
Testing models
29 Sep 2022
Only used the base
model so far.
Testing medium.en
now.
1.42Gb download.
Getting now a ValueError(f"This model doesn't have language tokens so it can't perform lang id")
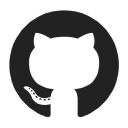
testing long file
01 Oct 2022
With Tesla's AI Day online since today, I wanted to get a transcript of it.
Video file downloaded from Youtube is 14Gb.
Extracted audio-only How to extract audio from video on macOS resulting in a 197Mb .m4a
file.
Good news:
Whisper transcribed it in 133 minutes with settings as:
model = whisper.load_model("medium")
response = model.transcribe(file_path,language='english')
Bad news:
152,288 characters on 7 lines!
Hope to see improvements re layout and speaker recognition in future updates.
Will check tomorrow for accuracy & SRT implemenentation.
04 Oct 2022
SRT generation implemented with:
from whisper.utils import write_srt
model = whisper.load_model("medium")
response = model.transcribe(file_path,language='english')
# text = response["text"]
# language = response["language"]
srt = response["segments"]
parent_folder = os.path.abspath(os.path.join(file_path, os.pardir))
# write SRT
with open(f"{parent_folder}/{uid}.srt", 'w', encoding="utf-8") as srt_file:
write_srt(srt, file=srt_file)
Again, works surprisingly well 😁
See example with a 3.5h video (transcribed in 2h) at Tesla AI Day 2022
Command line
Can be used as
whisper audio_or_video_file.m4a --language en --model medium
Resources
Available models & languages:
Size | Parameters | English-only model | Multilingual model | Required VRAM | Relative speed |
---|---|---|---|---|---|
tiny | 39 M | tiny.en |
tiny |
~1 GB | ~32x |
base | 74 M | base.en |
base |
~1 GB | ~16x |
small | 244 M | small.en |
small |
~2 GB | ~6x |
medium | 769 M | medium.en |
medium |
~5 GB | ~2x |
large | 1550 M | N/A | large |
~10 GB | 1x |
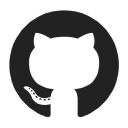
Enhancing results:
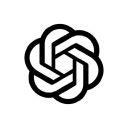
Mac Apps
15 Mar 2024
Available now in Setapp: superwhisper - testing now.
Otherwise this seems to be the best?
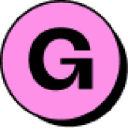